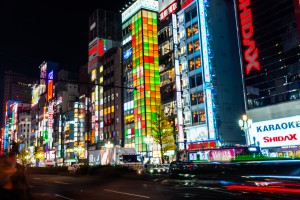
In the innovations that are considered as promised to revolutionize our World, artificial intelligence is often ranked at the top of the list. Whether it be Deloitte, Bloomberg, or many other analysis or media firms, artificial intelligence is considered as the most disruptive technology at the moment.
In this way, pharmaceutical laboratories, GAFA and other startups have entered the breach through projects to decrypt the human genome, creation of new molecules for the pharmaceutical industry, or even recruiting patients for clinical trials, there is no lack of examples…
The pharmaceutical world is far from being the only one, and recently all sectors seem to take the latest artificial intelligence technologies to conduct integration tests in their business process.
At the time when artificial intelligences have defeated the best Go players in the World, when they learned to play chess alone, with no help other than the rules, and here again, in a few hours of prior learning, beaten the best, at the time when they have learned to “reproduce”, when they created new languages by talking to each other, and when they have, in just two years, reached the IQ of 47… At this moment, it is important to ask what the future of these technologies is for the monitoring and competitive intelligence businesses.
Artificial intelligence and information monitoring
With hindsight that I think I have on the job better in an extremely pragmatic way, I identify a set of tasks rather time-consuming, and not all really interesting, for which we are entitled (we must even) ask about the usefulness of artificial intelligence to replace human beings.
Automatic translation
Within the useful tasks that the watcher might have to renounce, we find translation. When we monitor the web, linguistic knowledge is a real asset. If English seems to be an imperative for any watchers because of the importance of the English language in scientific publications, business, and more generally in the mass of published information, other languages are often hard to integrate into the monitoring process because of linguistic skills and/or costs.
Although automatic translation tools such as Systran or Google Translate can help, they will not manage to reach a certain degree of finesse of understanding and will not allow translation of documents for “clean” official publications without going through human translation. Google has also understood that perfectly and offers its community of users to perform manual translations to enrich its translation database through its “community” site. The complexity and diversity of languages, their structures, require programming and/or specific training for translation-oriented artificial intelligences which until now represented a significant cost.
However, the investment in this field, of the major players of the Web, allowed for a colossal progress in terms of translation quality on a planetary scale. It should be noted that since August 2017, the translations provided by Facebook are entirely done by artificial intelligences, offering with respect to the previous translation system, an improvement in the quality of the translation of 11% by relying on neural networks able to have a short-term memory, to treat longer word sequences together, to “cope” with words it does not know…
The latest innovation to date, the launch on the market of a simultaneous translation headset. Dernière innovation en date, la mise sur le marché d’une oreillette de traduction simultanée.
Cleaning and structuring information
When browsing a web page, an online press article, the human eye can tell the difference between the article’s text and all the peripheral content. It can also quickly assign a date to the article as well as the author when the information is present.
For any watcher, the inability of current tools to complete these basic tasks for human being, results in time-consuming and unattractive asks of verification and meta data qualification, but also information “cleaning” tasks. Whether it be banners, menus, or more and more polluting elements integrated in the middle of the article, the watcher will lose a lot of time cleaning the information.
The inability of monitoring tools (Editor’s note: most of the tools, I will not mention any name) to carry out these tasks often pollute the flow of information. When the task of “zoning” interesting information occurs too late in the extraction and filtration process, the key words present in the peripheral zones (header, menu, footer, pubs, “To read”, Headlines, etc.) will pollute the flow.
The artificial intelligence tools are beginning to address these issues, Diffbot for example, offers APIs for such services (and we can also find the Spotlight Mercury API which is recommended by the late Readability, which was an example in terms of targeted articles extraction) but, while these performances are interesting for press articles, they stay average for online scientific articles, for example. Besides, these artificial intelligence tools rely without always mentioning it, on the analysis of code, of templates and, of “objects” within the source code of web pages. Without knowledge or prior learning of the model or the “objects”, the AI will often fail.
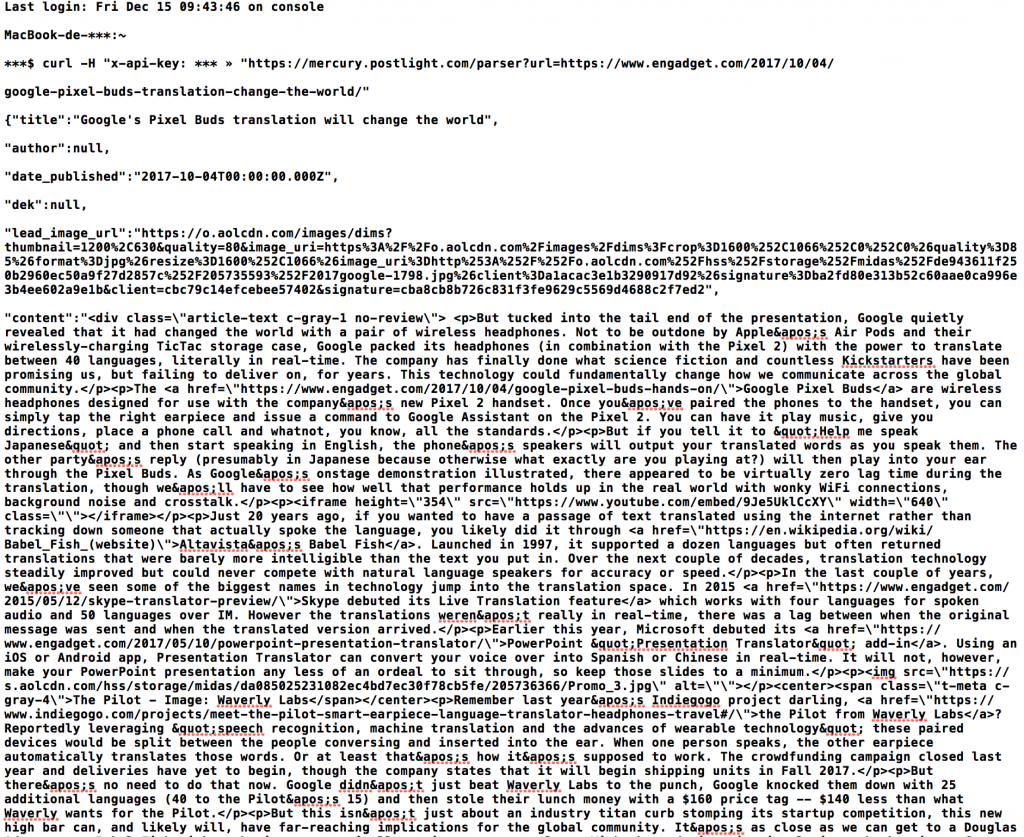
Above the result of the automatic extraction of an article via the API proposed by Mercury.
To date, however, we note relatively few (if any) projects with artificial intelligence for these types of function and even fewer solutions which implement it in a complete process of crawling, structuring, filtering and publishing.
Understanding of the constituents of a theme
In the monitoring field, one of the fundamental tasks to translate the need of a customer, in front of the information existing online, in words and in research equations to capture all the necessary information and only that one.
This requires an understanding of the subject, its lexical field, by a confrontation with reality in the way information is put online by the stakeholders of the subject.
Needless to say, Google seems to be the one that looked at this topic the most with its “rank brain” algorithm. The development of digital assistants like Siri, Google Home, Alexa and others, allows also the joint construction of evolving, contextualized and personalized thesauri, whose goal is to understand language, including questions. Progress being made is however made on tasks and factual and operational questions, and not on lexical understanding related to broad areas of knowledge with fuzzy or abstract boundaries.
While clear progress is already visible between the broadcast by a searcher/watcher and the understanding of his machine, we still need a lot of human work to build the set of requests to best cover an area of investigation.
Tone
The Holy Grail of the communicator, knowing the negative and positive views and opinions on a product, a brand. Let’s not deceive ourselves, the technology is bad enough on this point. Ambiguity of language, irony, elision of certain subjects, obvious confusion on sectors of activity denoted negatively in the absolute (humanitarian, for example), the moderately effective algorithms to tell you if the article is positive or negative concerning your subject.
Some social media listening solutions such as Crimson Hexagon, ahead of their competitors, had integrated machine learning, offering the user the ability to manually qualify a certain number of messages to help it “understand”. Today we have to look at APIs from the text analytics sector to find most powerful solutions such as Lexalytics’ Semantria.
Here again, the progress margin is colossal…
The discovery of relevant sources
Each watcher is well acquainted with the fundamental task of sourcing, which consist in constituting a bundle of sources producing potentially interesting information on a defined monitored theme. Ideally, watchers would monitor the web in its entirety, but to this day, it is Google that comes closest to an infrastructure capable of indexing the global web, and it has a colossal infrastructure for that. The watcher and the monitoring software editors must deal with it, in the monitoring process, the sourcing corresponds to a fundamental step, allowing to monitor priority sources in direct correlation with technical constraints and time constraints (of information treatment).
In monitoring software publishers, it is, to my knowledge, IXXO (web mining) that has developed the most technologically advanced exploratory crawl solution, the software starts from priority crawl points, and crawls deep links (internal and external links) and thus finds information, and also sources related to a theme.
Outside this software solution, few players of the sector offer a real module to help source discovery if it is not to offer their own source packages (often relying on the publisher’s internal sourcing pooled with the sourcing done by their customers) and a possible “Google” module, the software relying on queries sent to the famous search engine to find its latest news.
The margin progress is also huge from this perspective… By relying on sites such as Reddit, Twitter, Google News, or any other republishing and information sharing tools, by coupling a conditional and semantic crawler, by developing efficient tools recognizing interesting text sections and detecting dates, and finally by adding a learning machine or even an artificial intelligence module to learn with the user what is an interesting source to include in the monitoring project, the functionality could simply be revolutionary for our trades. A self-learning monitoring perimeter that would detect and correct dead links, learn to adjust its extraction settings and lastly, offer the addition of semi-automatic sources. It is not forbidden to dream.
The future of our business facing artificial intelligence
At this stage of progress of artificial intelligence technologies, it seems to me that the job of analyst is far from being questioned. The ability of artificial intelligence to synthesize complex and sometimes abstract subjects remains today limited, but it also remains limited because the human being still struggles to model and formalize the process of intellectual analysis that relies on sometimes diffuse knowledge, unconscious perceptions, and synthesis and complex information treatment processes to set it to music.
However, it seems to me that the job of watcher and the positioning “setting/selection/validation” could potentially and up in a delicate position (even more delicate than that of today). If today the latter is globally saved by the precariousness of the monitoring software business model whose business capital is globally incompatible with a strict respect of copyrights, the major players in digital information have redoubled their investments these last years to develop new semantic technologies and artificial intelligence.
Finally, I can only advocate so that the job of the watcher becomes more technician or more analyst.
During my last years of work, I noticed a strong scanning and digitization of the tools. The process has been going on for a long time, of course, but we can see how much it has accelerated. Today, it clearly falls within the key abilities to master certain fundamentals related to digital information and its access: web mining, API, text mining, etc.
In the end, I have seen over the years the number of watchers depletes, they often became analysts with dual monitoring + sectoral expertise (FRENCH). Watchers have increasingly evolved towards functions that lead them to (pre) analyze information and provide a qualified, digested, simplified, enriched version.
And you? What is your opinion on artificial intelligence and its contribution to our professions? Which interesting readings or articles have you noticed?
Photo credits: Frédéric Martinet – Any reproduction or use prohibited without prior agreement